Next: Varianz und höhere Momente
Up: Momente von Zufallsvariablen
Previous: Momente von Zufallsvariablen
  Contents
Erwartungswert
Bevor wir zur allgemeinen Definition des Erwartungswertes kommen,
wollen wir die intuitive Bedeutung dieses Begriffes anhand des
folgenden Beispiels erläutern.
- Beispiel
(wiederholtes Würfeln)
- Betrachten den Wahrscheinlichkeitsraum
mit der Grundmenge
und dem Wahrscheinlichkeitsmaß
, das durch
gegeben ist;
;
;
.
- Betrachten die Zufallsvariablen
, die gegeben seien durch die
Projektion
für
.
D.h.,
ist die (zufällige) Augenzahl, die beim
-ten Würfeln erzielt wird.
- Es ist nicht schwierig zu zeigen,
daß
unabhängige (und identisch verteilte)
Zufallsvariable sind.
- Betrachten die Zufallsvariable
, d.h. die mittlere Augenzahl
bei
-maligem Würfeln.
- Man kann zeigen, daß es eine ,,nichtzufällige'' Zahl
gibt,
so daß
 |
(1) |
wobei
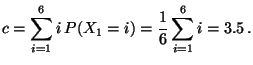 |
(2) |
- Die Formeln (1) und (2) bedeuten:
Falls die Anzahl
der durchgeführten Versuche immer größer
wird, dann
- werden die Werte
der mittleren Augenzahl
immer weniger
von der jeweiligen Ausprägung
des Zufalls beeinflußt,
- strebt das ,,Zeitmittel''
bei
Versuchen gegen das
,, Scharmittel'' c jedes (einzelnen) Versuches.
- Die Formeln (1) und (2) sind
ein Spezialfall des sogenannten Gesetzes
der großen Zahlen, das im weiteren Verlauf der Vorlesung noch
genauer diskutiert wird.
- Das Scharmittel
in (2) wird Erwartungswert der
Zufallsvariablen
genannt und mit
bezeichnet.
Auf analoge Weise wird der Begriff des Erwartungswertes für
beliebige (diskrete bzw. absolutstetige) Zufallsvariable
eingeführt.
- Definition 4.1
Betrachten einen beliebigen
Wahrscheinlichkeitsraum
.
- Sei
eine diskrete Zufallsvariable
mit
für eine abzählbare Menge
. Dann heißt das gewichtete Mittel
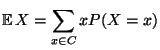 |
(3) |
der Erwartungswert von
,
wobei vorausgesetzt wird, daß
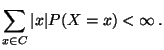 |
(4) |
- Sei
eine absolutstetige Zufallsvariable
mit der Dichte
. Dann heißt das Integral
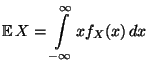 |
(5) |
der Erwartungswert von
, wobei vorausgesetzt wird, daß
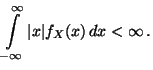 |
(6) |
- Beachte
-
- Beispiele
Wir zeigen nun anhand zweier Beipiele, wie
die Formeln (3) und (5) zur Bestimmung des
Erwartungswertes genutzt werden können.
- Binomialverteilung
Sei
binomialverteilt mit den Parametern
und
. Dann ergibt sich aus (3), daß
- Normalverteilung
Sei
normalverteilt mit den
Parametern
und
.
Dann ergibt sich aus (5), daß
- Beachte
-
Next: Varianz und höhere Momente
Up: Momente von Zufallsvariablen
Previous: Momente von Zufallsvariablen
  Contents
Roland Maier
2001-08-20