Next: Coupling Algorithms; Perfect MCMC
Up: Error Analysis for MCMC
Previous: MCMC Estimators; Bias and
  Contents
Asymptotic Variance of Estimation; Mean Squared Error
For the statistical model introduced in Section 3.4.2
we now investigate the asymptotic behavior of the variance
if
.
Theorem 3.19

Define
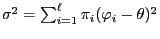
and let
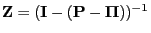
be the fundamental matrix of

defined by

. Then
 |
(78) |
- Proof
-
- Clearly,
 |
(79) |
and thus
- This representation will now be used to show (78) for
the case
.
- In this case we observe
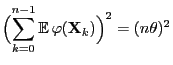
and
- Furthermore, by the stationarity of the Markov chain
,
where
and
denotes the matrix of
the
-step transition probabilities.
- A combination of the results above yields
- where the second equality is due to the identity
- Taking into account the representation formula (76)
for
this implies (78).
- It is left to show that (78) is also true for an
arbitrary initial distribution
.
- Remarks
-
In order to investigate this problem more deeply we introduce the
following notation: Let
where
is an arbitrary function and
is an arbitrary reversible pair.
- Proof
-
- Let
be a transition matrix such that the pair
is reversible. It suffices to show that
 |
(83) |
- By Theorem 3.19,
 |
(84) |
where
denotes the fundamental matrix of
introduced
by (74).
- On the other hand, as
, we get that
and thus
- Taking into account (84) this implies
 |
(85) |
- As the pair
is reversible, by the representation
formula (75) for the fundamental matrix
that was derived in Lemma 3.3 we
obtain for arbitrary
- This implies
- Thus, by (85),
 |
(86) |
where the last equality is due to the fact that
which is an immediate consequence of the definition
(74) of
.
- As
is a stochastic matrix and
is
reversible
- This completes the proof of (83).
- Remarks
As a particular consequence of Theorem 3.20 we get
that
- the simulation matrix
of the Metropolis algorithm (i.e.
if we consider equality in (50) ) minimizes the
asymptotic variance
- within the class of all Metropolis-Hastings algorithms having an
arbitrary but fixed ,,potential transition matrix''
.
Next: Coupling Algorithms; Perfect MCMC
Up: Error Analysis for MCMC
Previous: MCMC Estimators; Bias and
  Contents
Ursa Pantle
2006-07-20