Nächste Seite: Maximum-Likelihood-Gleichung und numerische Lösungsansätze
Aufwärts: Maximum-Likelihood-Schätzer für
Vorherige Seite: Loglikelihood-Funktion und ihre partiellen
  Inhalt
Hesse-Matrix
Neben dem (Score-) Vektor
der ersten partiellen
Ableitungen der Loglikelihood-Funktion
wird auch ihre Hesse-Matrix, d.h., die
-Matrix
der zweiten partiellen Ableitungen benötigt.
Theorem 4.2
- Für jedes GLM gilt
 |
(34) |
wobei
die in
gegebene
Fisher-Informationsmatrix und
eine
-Diagonalmatrix ist mit
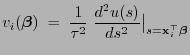
und
- Für GLM mit natürlicher Linkfunktion gilt insbesondere
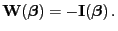 |
(35) |
- Beweis
-
- Aus Formel (27) in Theorem 4.1 ergibt
sich, dass für beliebige
- Dabei ergibt sich mit der Schreibweise
aus Lemma 4.2, dass
und somit
- Außerdem gilt
- Insgesamt ergibt sich also, dass
- Hieraus und aus der Darstellungsformel (29) für die
Fisher-Informationsmatrix
ergibt sich
(34).
- Weil für GLM mit natürlicher Linkfunktion die Superposition
die Identitätsabbildung ist, gilt in diesem Fall
. Somit ergibt sich (35)
aus (34).
- Beachte
Für die Beispiele von GLM, die in
Abschnitt 4.2 betrachtet worden sind, ergeben sich
aus den Theoremen 4.1 und 4.2 bzw. aus
Korollar 4.1 die folgenden Formeln für
und
.
- 1.
- Für das lineare Modell
mit normalverteilten
Stichprobenvariablen (und mit der Linkfunktion
) ist
die Einheitsmatrix. Somit gilt
 |
(36) |
vgl. auch Abschnitt 2.2.
- 2.
- Für das logistische Regressionsmodell (mit der
natürlichen Linkfunktion) gilt
 |
(37) |
wobei
und die
Wahrscheinlichkeiten
so wie in (20) durch
ausgedrückt werden können.
- 3.
- Für Poisson-verteilte Stichprobenvariablen mit
natürlicher Linkfunktion gilt
 |
(38) |
wobei
und
.
Nächste Seite: Maximum-Likelihood-Gleichung und numerische Lösungsansätze
Aufwärts: Maximum-Likelihood-Schätzer für
Vorherige Seite: Loglikelihood-Funktion und ihre partiellen
  Inhalt
Hendrik Schmidt
2006-02-27